Load Combinations#
Pystra includes the class LoadCombination
which can be used to analyse a structural reliability problem for different load cases. This example demonstrates the use of load combination cases with Pystra.
Example#
This demonstration problem is adopted from Example 4 pp 190-1 from Sorensen, J.D. (2004), Notes in Structural Reliability Theory And Risk Analysis. The parameter values are slightly modified for demonstration.
Import Libraries#
[1]:
import pystra as ra
import numpy as np
import pandas as pd
from matplotlib import pyplot as plt
Define the limit state function#
The LSF to be supplied in LoadCombination
can be any valid Pystra LSF. Here we show how random variables and even constants (e.g. cg
) can be passed through as Pystra objects, or can be hard-coded into the LSF.
[2]:
def lsf(R, G, Q1, Q2, cg):
return R - (cg*G + 0.8*Q1 + 0.2*Q2)
Define the Load and Resistance distributions#
We define the distributions of the loads for the load combinations. Some loads will be time-invariant (e.g. permanent loads), while some will be combination random variables (e.g. winds, imposed loads). These combination loads require the definition of a point-in-time ('pit'
) distribution and a maximum ('max'
) distribtribution (typically annual maximum). Note that Pystra has built in distribution objects to convert between the two: MaxParent
and Maximum
.
For the combination loads, we define a dictionary which contains a key for each variable in the LSF, and a dictionary with the pit
and max
key values being the corresponding Pystra distribution objects.
Define the annual max distributions
[3]:
Q1max = ra.Gumbel("Q1", 1, 0.2) # Imposed Load
Q2max = ra.Gumbel("Q2", 1, 0.4) # Wind Load
Next specifcy the parameters of inferred point-in-time parent distributions
[4]:
Q1pit = ra.Gumbel("Q1", 0.89, 0.2) # Imposed Load
Q2pit = ra.Gumbel("Q2", 0.77, 0.4) # Wind Load
Q_dict = {'Q1': {'max': Q1max, 'pit': Q1pit},
'Q2': {'max': Q2max, 'pit': Q2pit}}
And define any constants to be passed through
[5]:
cg = ra.Constant("cg", 0.4)
Finally, define any other random variables in the problem
[6]:
Rdist = ra.Lognormal("R", 2.0, 0.15) # Resistance
Gdist = ra.Normal("G", 1, 0.1) # Permanent Load (Other load variable)
Specify load combination cases#
For this problem, in addition to the permanent loads, we’re interested in investigating the reliabilities for several load combinations:
Q1Q2_max
: Where bothQ1
andQ2
maximum distributions are considered simultaneously, as an obvious conservative estimation.Q1_max
:Q1
is maximum andQ2
is the point-in-time distribution.Q2_max
:Q1
is the point-in-time distribution andQ2
is maximum.
We define a dictionary with the relevant loadcase names and corresponding variable names form the LSF.
[7]:
loadcombinations = {'Q1Q2_max':['Q1', 'Q2'], 'Q1_max':['Q1'], 'Q2_max':['Q2']}
Note: The specification of this dictionary is optional. By default, LoadCombination
assumes one combination load to act at its maximum in a load case, while the others are considered to be the point-in-time distribution.
Specify user-defined correlation (optional)#
The user can also specify a user-defined correlation matrix for the random variables of the reliability problem as a DataFrame.
[8]:
label_rvs = ["Q1", "Q2", "R", "G"]
corr = np.eye(len(label_rvs))
df_corr = pd.DataFrame(data=corr, columns=label_rvs, index=label_rvs)
corr_Q1Q2 = 0.8
df_corr.loc["Q1", "Q2"] = corr_Q1Q2
df_corr.loc["Q2", "Q1"] = corr_Q1Q2
print(df_corr)
Q1 Q2 R G
Q1 1.0 0.8 0.0 0.0
Q2 0.8 1.0 0.0 0.0
R 0.0 0.0 1.0 0.0
G 0.0 0.0 0.0 1.0
Specify user-defined analysis options (optional)#
The user can also specify a user-defined correlation Pystra AnalysisOptions
object for the reliability analysis too. In this tutorial we will set error thresholds and change the default transform method to use singular value decomposition.
[9]:
options = ra.AnalysisOptions()
options.setE1(1e-3)
options.setE2(1e-3)
options.setTransform("svd")
Instantiate LoadCombination
object#
LoadCombination
class requires specification of combination distributions as a dictionary, while the other distributions (permanent loads and resistances) are specified as a list.
[10]:
lc = ra.LoadCombination(lsf=lsf, dict_dist_comb=Q_dict, list_dist_resist=[Rdist], list_dist_other=[Gdist],
corr=df_corr, list_const=[cg], opt=options, dict_comb_cases=loadcombinations)
Analyse Load Cases#
Use a list to keep track of each Form
object outputted:
[11]:
form = 3*[None]
Load Case 1#
[12]:
lc_idx = 0
form[lc_idx] = lc.run_reliability_case(lcn='Q1Q2_max')
form[lc_idx].showDetailedOutput()
======================================================
FORM
======================================================
Pf 2.5348733315e-02
BetaHL 1.9540317148
Model Evaluations 38
------------------------------------------------------
Variable U_star X_star alpha
R -1.818038 1.898248 -0.930293
G -0.659741 1.018495 -0.337834
Q1 0.184954 1.423639 +0.095051
Q2 -0.208597 1.759687 -0.106715
cg --- 0.400000 ---
======================================================
Load Case 2#
[13]:
lc_idx = 1
form[lc_idx] = lc.run_reliability_case(lcn='Q1_max')
form[lc_idx].showDetailedOutput()
======================================================
FORM
======================================================
Pf 1.9624337657e-02
BetaHL 2.0615701821
Model Evaluations 49
------------------------------------------------------
Variable U_star X_star alpha
R -1.926554 1.896315 -0.934503
G -0.673345 1.018964 -0.326631
Q1 0.189636 1.461699 +0.091993
Q2 -0.221604 1.596853 -0.107490
cg --- 0.400000 ---
======================================================
Load Case 3#
[14]:
lc_idx = 2
form[lc_idx] = lc.run_reliability_case(lcn='Q2_max')
form[lc_idx].showDetailedOutput()
======================================================
FORM
======================================================
Pf 1.5511691106e-02
BetaHL 2.1567726618
Model Evaluations 49
------------------------------------------------------
Variable U_star X_star alpha
R -2.022994 1.894827 -0.937968
G -0.683828 1.019274 -0.317075
Q1 0.192740 1.386755 +0.089372
Q2 -0.233228 1.888565 -0.108134
cg --- 0.400000 ---
======================================================
Display reliability per load combination#
[15]:
for lc,β in zip([k for k in loadcombinations.keys()], [f.beta for f in form]):
print(f"{lc}: β = {β[0]:.2f}")
Q1Q2_max: β = 1.95
Q1_max: β = 2.06
Q2_max: β = 2.16
Distributions for Load Combinations#
Pystra
includes a range of tools to enable the calculation of load combinations due to variable loads:
Zero-Inflated Distributions allow for a probability of non-occurence of a load
Maximum Distributions allow the distribution of maxima of a supplied parent or point-in-time distribution
MaxParent Distribution allows the determination of the point-in-time distribution from a supplied maximum.
Zero-Inflated Distribution#
[16]:
zin = ra.ZeroInflated('Q',ra.Gumbel("G", 0.5, 0.2),p=0.9)
zin.zero_tol = 1e-2
x = np.linspace(-0.5,1.5,1000)
pdf = zin.pdf(x)
cdf = zin.cdf(x)
u = np.linspace(0,1,10001)
ppf = zin.ppf(u)
fig,axs = plt.subplot_mosaic([['a','c'],['b','c']],sharex=True)
ax = axs['a']
ax.plot(x,pdf)
ax.set_ylabel('$f(x)$')
ax.grid()
ax = axs['b']
ax.plot(x,cdf,label='Direct from CDF')
ax.plot(ppf,u,'r:',label='From Inverse CDF')
ax.set_ylabel('$F(x)$')
ax.set_xlabel('$x$')
ax.legend(loc='center right')
ax.grid()
ax = axs['c']
ax.plot(x,-np.log(-np.log(cdf)))
ax.set_ylabel('$-log[-log[F(x)]]$')
ax.set_xlabel('$x$')
ax.grid()
plt.tight_layout();
#fig.set_layout('tight');
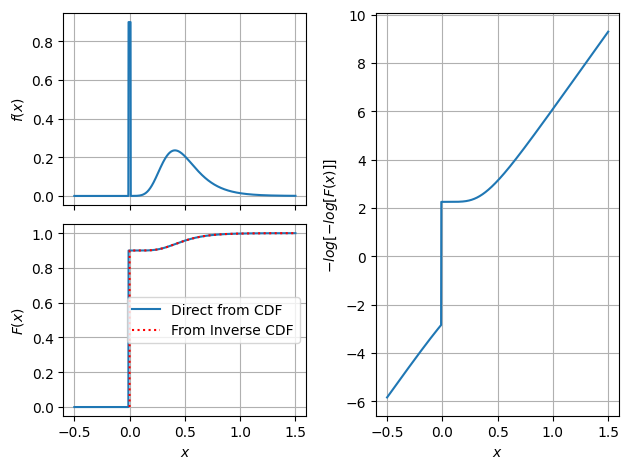
Example#
[17]:
def lsf(R,G,Q):
return R - (G + Q)
[18]:
def reliability(p=0.0):
ls = ra.LimitState(lsf)
sm = ra.StochasticModel()
sm.addVariable(ra.Lognormal("R", 6.0,0.15))
sm.addVariable(ra.Normal("G", 4.5, 0.2))
g = ra.Gumbel("Q", 0.5, 0.2)
if p == 1.0:
sm.addVariable(ra.Constant("Q",0.0))
elif p == 0.0:
sm.addVariable(g)
else:
zig = ra.ZeroInflated("Q",g,p=p)
sm.addVariable(zig)
form = ra.Form(sm,ls)
form.run()
return form
[19]:
betas = []
ps = np.linspace(0,1.0,101)
for p in ps:
form = reliability(p=p)
betas.append(form.beta)
[20]:
fig,ax = plt.subplots()
ax.plot(ps,betas,'r.:')
ax.set_xlabel('Probability of zero variable load, $p$')
ax.set_ylabel('Reliability index, $β$')
ax.grid();
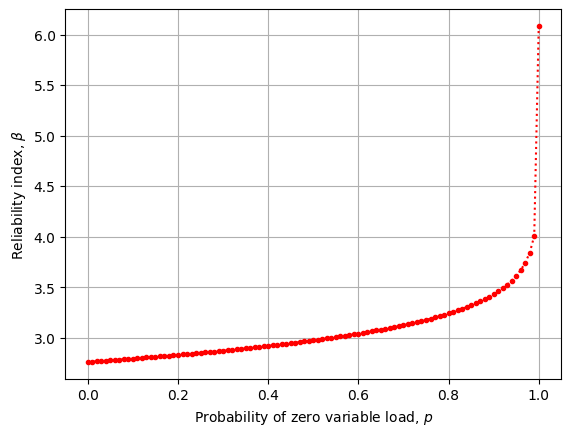